The advancement of optical fiber communication technology has taken a significant leap with the introduction of the Learnable Digital Signal Processing (LDSP) framework.
This innovative approach merges deep learning techniques with traditional digital signal processing modules, leading to highly effective signal optimization.
Research shows that LDSP can achieve a performance enhancement of up to 1.21 dB in Q factor, making it a game-changer in optical transmission.
LDSP not only reuses existing DSP modules but also optimizes them from a global perspective, paving the way for improved nonlinear compensation.
This framework holds promise for the future of optical communications, demonstrating notable improvements in both single and multi-channel transmission scenarios.
As researchers continue to explore its potential at conferences like IEEE, the implications for enhanced communication channels are profound.
While the LDSP framework offers impressive benefits, it is not without challenges.
Increased complexity may demand higher computational resources, and integrating this technology with existing optical systems could pose difficulties.
Nevertheless, the long-term benefits of adopting this deep learning-based approach could outweigh the initial hurdles, making it a crucial focus for the future of optical fiber technology.
Revolutionizing Optical Fiber Communication With LDSP
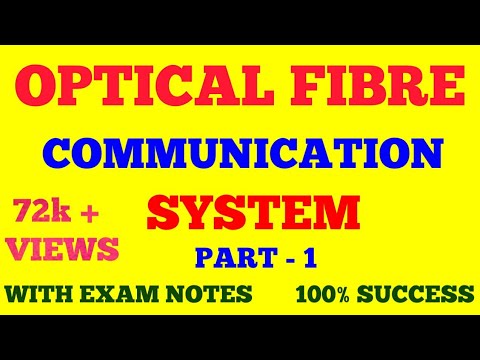
The integration of Learnable Digital Signal Processing (LDSP) is changing the landscape of optical fiber communication.
By combining deep learning with traditional DSP techniques, LDSP enhances signal performance and reduces complexity.
This approach leads to significant performance improvements, setting new benchmarks in the field.
Merging Deep Learning With Traditional DSP Techniques
LDSP creates a powerful synergy between deep learning methods and existing digital signal processing (DSP) frameworks.
This merging allows for the reuse of traditional DSP modules within a more advanced structure.
Using deep learning, researchers can optimize performance on a broader scale, achieving better results than conventional techniques alone.
This integration paves the way for enhanced signal processing capabilities, ensuring that both single and multi-channel transmissions benefit from improved outcomes.
Global Optimization Approach for Enhanced Signal Processing
The LDSP framework employs a global optimization strategy that focuses on overall system performance.
This approach moves beyond simple adjustments to specific components, which often limits performance gains.
By evaluating the entire communication system, it can fine-tune parameters that contribute to nonlinear compensation.
This results in measurable improvements, such as a 1.21 dB gain in Q factor, showcasing its effectiveness.
The global perspective fosters compatibility with future techniques, making LDSP a forward-thinking solution for optical fiber communications.
Overcoming Limitations of Conventional DSP Frameworks
One of the main advantages of LDSP is its potential to overcome the limitations present in traditional DSP frameworks.
Conventional methods often struggle with nonlinear effects that can degrade signal quality.
By implementing LDSP, researchers can address these challenges more effectively.
Although the increased complexity might raise computational demands, the long-term benefits include better signal performance and reduced implementation costs.
This shift signifies a move towards more reliable and efficient optical systems, even as the challenge of integrating these new frameworks with existing technology persists.
Experimental Validation and Performance Breakthroughs
The integration of the LDSP framework into optical fiber technology has led to significant advancements. Key improvements in transmission quality, nonlinear compensation, and the effectiveness of multi-channel communication highlight the transformative impact of this approach.
Significant Q Factor Improvements in Signal Transmission
Recent experiments have demonstrated that the LDSP framework can achieve up to a 1.21 dB gain in Q factor.
This enhancement results in better signal integrity and lower bit error rates.
The Q factor measures the quality of the signal transmitted, indicating how well it can be detected without interference.
Improved linearity compensation plays a crucial role here, allowing for more accurate reception.
By minimizing chromatic dispersion and polarization mode dispersion (PMD) effects, the receivers can now handle longer reach while preserving signal clarity.
These breakthroughs are vital for high-speed communication where clarity is paramount.
Impact on Single-Channel and Multi-Channel Communication
The LDSP framework significantly influences both single-channel and multi-channel communication.
By leveraging digital backpropagation and perturbation-based nonlinear compensation, the system optimizes signal processing across multiple channels.
Users can expect smoother data transmission and increased throughput in multi-channel settings.
The experiments confirm robust performance in diverse environments, tackling challenges such as nonlinear Schrödinger equations that govern light behavior in fibers.
This adaptability suggests that as networks grow in complexity, the LDSP framework can maintain high performance.
Enhancing Nonlinear Compensation in Optical Systems
The need for effective nonlinear compensation is increasingly critical as optical systems evolve.
LDSP utilizes advanced algorithms to tackle nonlinear effects encountered during transmission.
This system not only adjusts for polarization mode dispersion (PMD) but also enhances the effectiveness of traditional compensation methods.
By incorporating nonlinear estimation, the framework demonstrates superior capabilities over previous models, allowing for a more efficient response to signal distortions.
In practical applications, this means that systems can increase their capacity and reliability, helping to achieve a new benchmark in optical fiber linearity. Learn more about this development here.
Future Implications and Challenges in Optical Fiber Technology
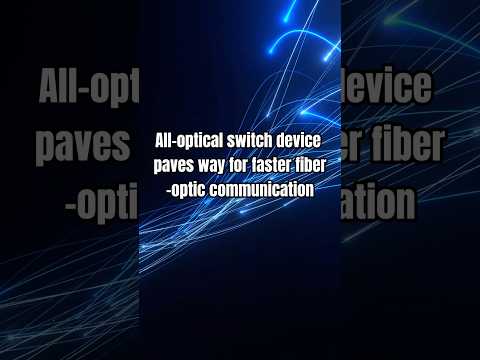
The advancements in optical fiber technology, particularly through the use of machine learning and deep learning, promise significant improvements in performance and efficiency.
However, along with the potential gains, there are challenges that need to be addressed for successful integration into existing systems.
Potential for Increased Transmission Capacity and Efficiency
With the application of Learnable DSP (LDSP), there is potential for enhanced transmission capacity and efficiency.
The LDSP framework employs deep neural networks and the backpropagation algorithm to optimize signal processing tasks.
This integration allows for real-time adjustments and improvements in transmission quality.
Experimental trials have shown an increase in the Q factor by up to 1.21 dB, indicating a notable enhancement in signal performance.
Such improvements can significantly benefit long-haul links, where maintaining signal integrity over vast distances is crucial.
This approach enables better nonlinear compensation, thus overcoming critical limitations of traditional DSP methods.
Addressing Implementation and Integration Challenges
Although LDSP shows great promise, its implementation poses challenges.
The increased complexity in signal processing may heighten computational requirements.
Integrating these advanced systems with existing optical frameworks can face obstacles due to legacy technologies that are not compatible with modern deep learning tools.
Another consideration is the cost of implementation. While the gains in performance could justify the investment, organizations must weigh these benefits against potential trade-offs.
Ensuring long-term reliability of these deep learning-based methods in practical applications is also a concern.
Stakeholders must address these issues to ensure that the benefits can be realized effectively.
Evolving Role of Machine Learning in Optical Communications Infrastructure
Machine learning is poised to revolutionize the optical communications infrastructure. By utilizing methods like stochastic gradient descent (SGD), systems can benefit from rapid adaptation and optimization processes.
This evolution enables optical networks to become smarter and more efficient.
Traditional DSP modules can be restructured within a machine learning framework to optimize performance from a global standpoint.
This shift not only facilitates enhanced nonlinear compensation techniques but also supports multi-channel transmission improvements.
The ongoing development of these systems indicates a future where optical fiber technology can achieve unprecedented levels of efficiency and capacity.