Recent advancements in optical fiber communication have introduced an innovative framework known as Learnable Digital Signal Processing (LDSP).
This technology combines deep learning with traditional digital signal processing to address key challenges in optical communications.
The LDSP framework significantly enhances signal performance and improves nonlinear compensation, making it a game-changer in the field of optical fiber technology.
With its unique approach, LDSP optimizes the performance of optical communication systems by treating the entire process as a deep learning structure.
This adaptation not only reuses existing DSP modules but also paves the way for better performance through comprehensive module-level optimization.
Experimental trials reveal that the framework can achieve an impressive gain in Q factor for single-channel transmission, which directly impacts signal clarity and integrity.
While the LDSP framework showcases remarkable potential, it does come with considerations.
The complexity of this system may increase computational demands, and integration with current technologies could pose challenges.
Balancing the benefits against implementation costs remains crucial for long-term success in the optical fiber landscape.
As the industry looks toward the future, LDSP represents a vital step toward optimizing high-capacity and reliable optical fiber communication.
Understanding The LDSP Framework In Optical Fiber Communication
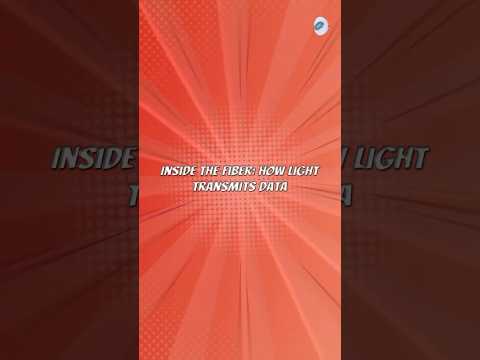
The LDSP framework represents a significant advancement in optical fiber communication. It merges deep learning techniques with traditional digital signal processing (DSP) methods to enhance signal performance and improve nonlinear compensation.
Integration Of Deep Learning With Traditional DSP Modules
The LDSP framework reuses existing DSP modules while incorporating deep learning elements. This integration treats the whole signal processing task as a deep learning structure.
Using techniques like the backpropagation algorithm and stochastic gradient descent, the framework optimizes the performance of these traditional modules.
This blending allows for precise adjustments in processing techniques, leading to improved signal clarity and quality.
As a result, the optical fiber communication systems demonstrate better handling of nonlinearity issues, a common challenge in high-speed data transmission.
Global Optimization Approach For Enhanced Performance
The LDSP framework adopts a global optimization strategy to enhance performance across various dimensions.
By examining the entire communication system holistically, it employs hyperparameter optimization techniques to refine settings for digital signal processing.
This method identifies optimal arrangements that boost the quality factor (Q factor) of the signal.
Experimental results show gains of up to 1.21 dB in Q factor for single-channel transmission. Such improvements indicate that the framework can significantly boost performance while maintaining low complexity in DSP designs.
Compatibility With Future Nonlinear Compensation Techniques
The LDSP framework is designed with future advancements in mind. Its structure supports compatibility with upcoming nonlinear compensation methods, paving the way for more efficient optical systems.
The approach ensures that as new nonlinear DSP designs emerge, they can be integrated seamlessly within the existing framework.
This adaptability is vital for the evolving landscape of optical fiber communications.
As researchers continue to develop better nonlinear estimation techniques, the LDSP framework stands ready to incorporate these innovations, further enhancing optical fiber performance in the future.
Experimental Validation And Performance Improvements
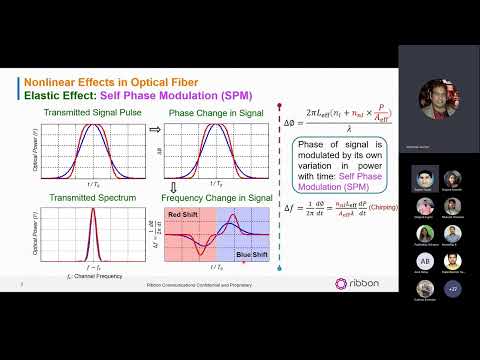
The implementation of the Learnable Digital Signal Processing (LDSP) framework has led to impressive changes in signal performance and nonlinear compensation in optical fiber technology.
Experimental results indicate notable increases in key metrics like the Q factor and improvements in both single-channel and multi-channel transmissions.
Significant Enhancements In Q Factor For Signal Performance
Trials have shown that LDSP achieves a remarkable increase in the Q factor, which is crucial for evaluating signal quality.
This framework demonstrated a gain of up to 1.21 dB in Q factor for single-channel transmission. The improvement correlates with reduced bit error rates and enhanced signal-to-noise ratios.
Higher Q factors mean that signals can be transmitted more reliably through fiber transmission systems.
By optimizing performance against linear distortions and chromatic dispersion, LDSP enhances the overall performance of coherent optical systems, allowing for clearer signal communication.
Impact On Single-Channel And Multi-Channel Transmission
LDSP significantly impacts both single-channel and WDM transmissions (wavelength division multiplexing).
In single-channel scenarios, enhanced power levels and reduced ASE noise lead to clearer signals and better performance.
For multi-channel systems, LDSP effectively manages the complexities involved in transmitting signals simultaneously over various channels.
This results in improved transmission performance, ensuring that signals maintain integrity even with increased traffic and potential fiber nonlinearities.
Furthermore, LDSP’s application reduces the adverse effects of linear impairments commonly encountered in high-performance optical systems.
Comparative Analysis With Traditional DSP Frameworks
When compared to traditional digital signal processing methods, LDSP stands out for its ability to integrate deep learning techniques.
This modernized approach allows for comprehensive optimization of existing DSP modules, addressing both efficiency and accuracy.
In contrast, traditional frameworks often struggle to accommodate the increasing demands for high performance under challenging transmission conditions.
LDSP’s method of treating the signal process as a holistic learning structure facilitates substantial enhancements in the performance of optical systems while maintaining compatibility with existing setups.
Such comparative advantages underline the potential of the LDSP framework to reshape future standards in optical fiber technology and communication systems, as highlighted in the New Benchmark for Optical Fiber Linearity Unveiled.
Future Implications And Challenges In Optical Fiber Technology
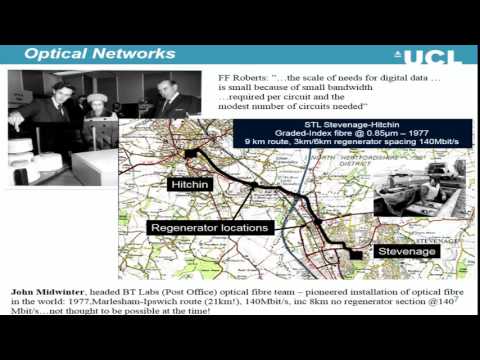
The advancement of optical fiber technology, particularly through frameworks like Learnable DSP (LDSP), brings both exciting possibilities and notable challenges.
As transmission capacity increases and new methods emerge, it is crucial to address the practical aspects of implementation and the evolving role of machine learning.
Potential For Increased Transmission Capacity In Optical Systems
Innovations in optical fiber technology, including LDSP, offer the potential for significant increases in transmission capacity.
This framework enhances signal performance and supports effective nonlinear compensation, which is vital for long-haul communication.
By reusing traditional digital signal processing (DSP) modules within a deep learning structure, the LDSP framework optimizes performance at every level.
As a result, experiments have shown up to a 1.21 dB gain in Q factor for single-channel transmissions. This increase indicates that higher data rates can be achieved without sacrificing signal quality.
The industry can leverage these advancements to support the growing demand for bandwidth in various applications, from consumer internet to enterprise-level communications.
Addressing Implementation Challenges In Existing Infrastructure
While the LDSP framework shows promise, integrating it into existing infrastructure has its challenges.
The complexity inherent in LDSP may increase computational requirements, making it crucial to assess whether current systems can support these advancements without significant upgrades.
There may be potential trade-offs between performance gains and the costs associated with implementing new technologies.
Operators must evaluate the reliability and stability of these systems in the long term. Addressing these challenges will be central to ensuring that advancements translate into practical benefits for users.
Collaboration among industry stakeholders is essential to develop standards that facilitate smoother integration and minimize disruption.
Evolving Role Of Machine Learning In Optical Communications
Machine learning is becoming increasingly vital in optimizing processes within optical communications.
The LDSP framework demonstrates how deep learning can enhance traditional methods, leading to better signal processing and performance.
As optical systems evolve, machine learning can help reduce computational complexity while maintaining efficiency.
This means that systems can operate effectively with lower power consumption, which is essential for sustainability.
The future of optical fiber will likely see further integration of machine learning techniques, creating an environment where continuous improvement is possible.
This can address nonlinear issues in real-time, ensuring robust performance and adaptability to varying conditions in communication networks.