In the rapidly evolving world of technology, a groundbreaking advancement in photonic in-memory computing is set to accelerate artificial intelligence (AI) capabilities to new heights. Recent research reveals that this innovative method utilizes magneto-optical materials to achieve impressive performance metrics. The implications of this technology are significant, as it promises high-speed, low-energy, and durable memory solutions that can seamlessly integrate with current computing systems.
This photonic breakthrough addresses existing limitations in optical memory, particularly for AI processing. Researchers have demonstrated switching speeds of just nanoseconds with endurance reaching 2.4 billion cycles. By combining non-volatility and multibit storage, this technology could redefine how data is processed and managed in AI applications, making the prospect of light-speed computing a tangible reality.
While still in its early stages, the potential for photonic in-memory computing to transform AI systems is enormous. As it gains traction, this method could streamline data handling and enhance the performance of various applications, paving the way for a future where AI operates smarter and faster than ever before.
The Foundation of Photonic Computing
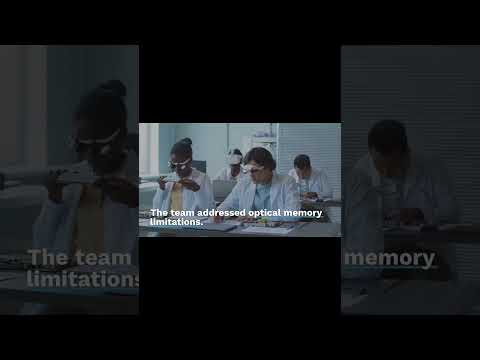
Photonic computing is an innovative approach that harnesses light for data processing and memory storage. This technology leverages the unique properties of photonics to address the limitations found in traditional electronic computing, especially in the realm of artificial intelligence.
The Role of Photonics in Computing
Photonics plays a crucial role in modern computing by using light instead of electricity to process data. This shift can lead to faster computation speeds and reduced energy consumption. Engineers are developing silicon-based photonic components that allow for efficient data transfer and processing.
One significant advantage of photonic systems is their ability to minimize heat production. Unlike traditional electronic circuits, which generate heat as electricity flows through wires, photonic systems maintain low temperatures, enabling them to handle large data volumes without overheating.
This technology primarily utilizes optical computing techniques, which signify a fundamental transformation in how information is processed. Researchers continue to explore photonic systems’ potential, aiming for wider applications in various fields, including AI and machine learning.
Photonic Memory and Data Processing
Photonic memory is a key area of focus in this burgeoning field. It offers several benefits over traditional memory solutions, like high-speed switching and low energy use. Recent advancements have demonstrated that photonic memory can achieve switching speeds measured in nanoseconds, allowing for rapid data access and processing.
One notable development involves using magneto-optical materials, such as cerium-substituted yttrium iron garnet, in conjunction with silicon micro-ring resonators. This combination provides a durable and efficient memory solution, capable of withstanding billions of switching cycles.
In-memory computing with photonics shows promise for combining storage and data processing in one step, enhancing efficiency and speed. As this technology matures, the integration with existing semiconductor technology will facilitate its practical deployment in various applications.
Advancements in AI Processing Technology
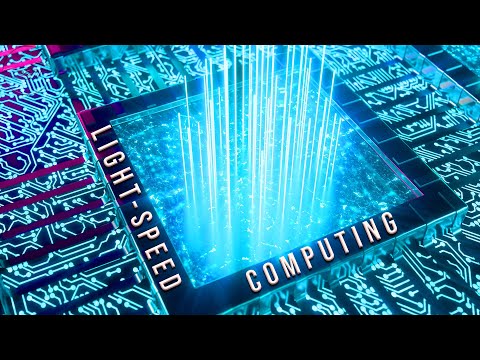
Recent developments in AI processing technology highlight significant improvements that enhance performance and efficiency. Key innovations in photonic in-memory computing and advancements in neural networks are paving the way for faster and more effective AI solutions.
Photonic In-Memory Breakthrough
Researchers have made strides in using magneto-optical materials to create photonic in-memory computing systems. This method combines non-volatility, multibit storage, and high switching speeds while consuming less energy. A notable example is the use of cerium-substituted yttrium iron garnet on silicon micro-ring resonators, enabling rapid data processing.
The breakthrough, published in Nature Photonics on October 23, 2024, demonstrates the technology’s capability, achieving an impressive 2.4 billion switching cycles at nanosecond speeds. This system is designed to work with existing complementary metal-oxide semiconductor (CMOS) technology, making it easier for companies to integrate into their current infrastructures. As researchers like Nathan Youngblood from the University of Pittsburgh explore scaling from individual cells to large arrays, this technology promises to overcome current limitations in optical memory related to AI.
Improving AI Training and Neural Networks
AI training relies heavily on processing vast amounts of data efficiently. Recent advances in computing technology directly support this need. The integration of photonic in-memory systems can lead to enhanced training of deep learning models and neural networks.
By utilizing the low energy and high speed of photonic systems, researchers can improve the performance of AI applications. This can result in faster training times and the ability to handle larger datasets. As demand for AI capabilities grows, these advancements are crucial for developing more sophisticated models.
Overall, improvements in memory speed and data processing efficiency allow AI technologies to evolve, paving the way for future innovations in various industries.
Seminal Research Contributions and Future Directions
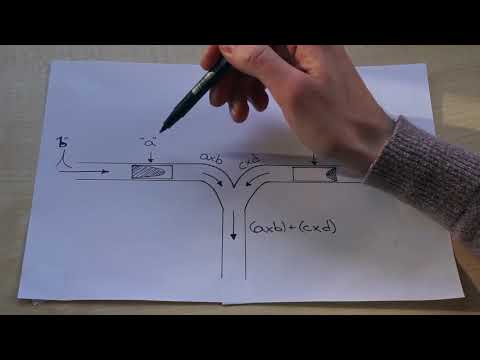
Recent breakthroughs in photonic in-memory computing indicate significant advancements in speed and efficiency for AI processing. Key contributions from various institutions highlight innovative methods and materials that enhance optical memory capabilities.
Impactful Studies and Research Outcomes
Researchers have developed a new photonic in-memory computing method using magneto-optical materials. This technology features high-speed, low-energy, and durable memory solutions. On October 23, 2024, a breakthrough study detailing these advancements was published in Nature Photonics.
An international team, including experts from the University of Cagliari and the Tokyo Institute of Technology, focused on enhancing optical memory in AI. They achieved impressive results, demonstrating 2.4 billion switching cycles at nanosecond speeds, which improves efficiency significantly. This technology is designed to integrate seamlessly with existing CMOS circuitry.
Future research will aim to scale these solutions from single memory cells to large memory arrays. Continued support from organizations like the National Science Foundation and the Air Force Office of Scientific Research will be critical in overcoming potential challenges in scaling and implementation.